Si trova su / Altri legami
© 2021 – IOS Press.Navigation apps have become more and more popular, as they give information about the current traffic state to drivers who then adapt their route choice. In commuting scenarios, where people repeatedly travel between a particular origin and destination, people tend to learn and adapt to different situations. What if the experience gained from such a learning task is shared via an app? In this paper, we analyse the effects that adaptive driver agents cause on the overall network, when those agents share their aggregated experience about route choice in a reinforcement learning setup. In particular, in this investigation, Q–learning is used and drivers share what they have learnt about the system, not just information about their current travel times. Using a classical commuting scenario, we show that experience sharing can improve convergence times that underlie a typical learning task. Further, we analyse individual learning dynamics to get an impression how aggregate and individual dynamics are related to each other. Based on that interesting pattern of individual learning dynamics can be observed that would otherwise be hidden in an only aggregate analysis.
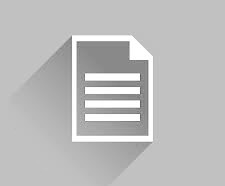