Si trova su / Altri legami
© 2021, The Author(s), under exclusive licence to Springer–Verlag GmbH, DE part of Springer Nature.We study multifrequency Hebbian plasticity by analyzing phenomenological models of weakly connected neural networks. We start with an analysis of a model for single–frequency networks previously shown to learn and memorize phase differences between component oscillators. We then study a model for gradient frequency neural networks (GrFNNs) which extends the single–frequency model by introducing frequency detuning and nonlinear coupling terms for multifrequency interactions. Our analysis focuses on models of two coupled oscillators and examines the dynamics of steady–state behaviors in multiple parameter regimes available to the models. We find that the model for two distinct frequencies shares essential dynamical properties with the single–frequency model and that Hebbian learning results in stronger connections for simple frequency ratios than for complex ratios. We then compare the analysis of the two–frequency model with numerical simulations of the GrFNN model and show that Hebbian plasticity in the latter is locally dominated by a nonlinear resonance captured by the two–frequency model.
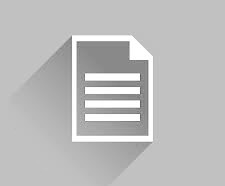